안녕하세요, 여러분!
오늘은 우리 일상에 조용히 혁명을 일으키고 있는
한 직업에 대해 이야기해볼까 합니다.
바로 '데이터 라벨링' 전문가인데요,
인공지능(AI)의 뒤에서
조용히 그러나 강력하게
AI를 지원하는 중요한 역할을 하고 있답니다.
데이터 라벨링이란 무엇일까요?
데이터 라벨링은 간단히 말해,
컴퓨터가 이해할 수 있도록
데이터에 '태그'나 '레이블'을 붙이는 작업입니다.
예를 들어, 사진 속 고양이를 '고양이'라고 태그하거나,
감정이 담긴 문장에 '기쁨', '슬픔' 등의
감정 레이블을 붙이는 것이죠.
이렇게 레이블을 붙인 데이터는
인공지능이 학습하는 교재와 같아요.
마치 어린아이에게 사물의 이름을 알려주듯,
AI에게 세상을 알려주는 역할을 합니다.
왜 중요할까요?
AI와 머신러닝의 세계에서 데이터는
'식량'과도 같습니다.
그리고 이 식량을 '소화'하기 쉽게 만드는 과정이
바로 데이터 레이블링이에요.
정확한 레이블링이 AI의 '영양 섭취'를 결정짓죠.
잘못된 레이블링은 AI를 '잘못된 길'로 인도할 수 있으니,
이 작업의 중요성을 결코 간과할 수 없습니다.
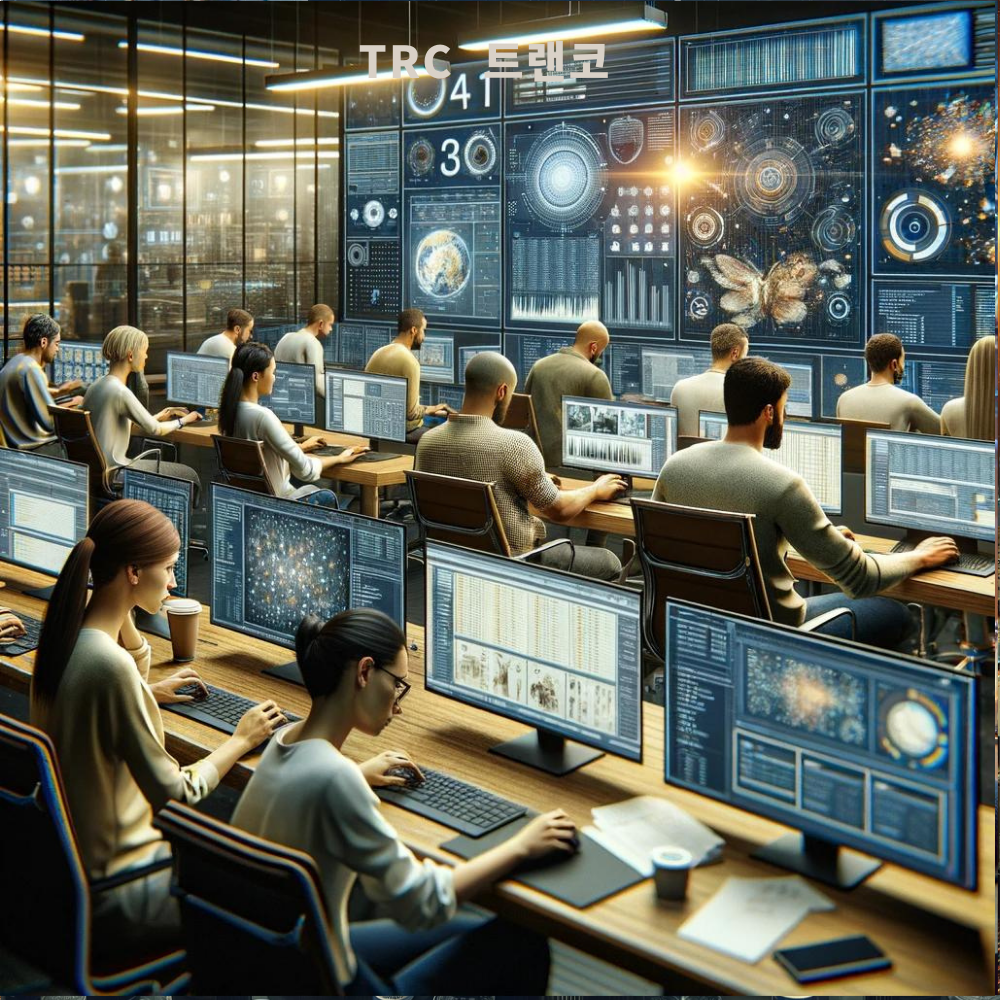
데이터 라벨링 전문가가 되려면?
데이터 라벨링은 특별한 배경 지식 없이도
시작할 수 있는 분야입니다.
하지만 섬세한 주의력과 정확성이 요구되죠.
이미지, 텍스트, 오디오 등
다양한 데이터 유형에 대한
기본적인 이해가 필요하며,
관련 도구와 소프트웨어를
능숙하게 다룰 수 있어야 합니다.
또한, AI와 머신러닝의 기본 개념을 이해한다면
더욱 효과적인 라벨링이 가능하겠죠.
미래의 전망은?
인공지능이 계속해서 발전함에 따라,
데이터 라벨링의 수요는
점점 더 증가할 것으로 예상됩니다.
이미 다양한 산업에서
AI의 도입이 확대되고 있으니,
데이터 레이블링 전문가로서의 경력도
매우 유망해 보입니다.

책추천
데이터 라벨링과 관련된 주제를 다루거나
데이터 과학, 머신러닝, 인공지능 분야에
도움이 될 수 있는 서적들을 추천해드립니다.
- "파이썬 머신러닝 완벽 가이드" - 권철민 지음
- 파이썬과 머신러닝 라이브러리를 활용한 실전 예제와 함께 데이터 분석 및 레이블링 기술을 배울 수 있는 책입니다.
- "핸즈온 머신러닝" - 오렐리앙 제롱 지음
- 실제 사례를 통해 머신러닝 프로젝트를 어떻게 진행하는지, 데이터를 어떻게 준비하고 레이블링하는지를 배울 수 있습니다.
- "밑바닥부터 시작하는 딥러닝" - 사이토 고키 지음
- 딥러닝의 기초부터 실전까지, 데이터를 어떻게 처리하고 학습시키는지에 대한 내용을 다루고 있습니다.
- "인공지능을 위한 수학" - 이시다 아키히코 지음
- 인공지능과 머신러닝에 필요한 수학적 기초를 알기 쉽게 설명해주는 책으로, 데이터 레이블링과 모델링에 필수적인 이론을 다룹니다.
- "데이터 과학을 위한 통계" - 라파엘 이라르트 지음
- 데이터를 이해하고 분석하는 데 필요한 통계적 개념을 설명하며, 데이터 레이블링에 있어서의 중요성을 강조합니다.
- "인공지능 비즈니스" - 조성준 지음
- 인공지능 기술과 비즈니스의 결합에 대해 다루며, 데이터 레이블링이 어떻게 비즈니스 가치를 창출하는지 설명합니다.
- "딥러닝을 이용한 자연어 처리 입문" - 유원준 지음
- 자연어 처리(NLP)에 초점을 맞추고, 텍스트 데이터 레이블링과 모델 학습 방법에 대해 설명합니다.
- "데이터 과학 프로젝트 핸드북" - 제이크 밴더플라스 지음
- 데이터 과학 프로젝트를 진행하는 과정에서 데이터 레이블링을 포함한 다양한 단계를 다루며, 실용적인 조언을 제공합니다.
- "인공지능의 미래" - 닉 보스트롬 지음
- 인공지능의 발전과 미래에 대해 탐구하며, 데이터 레이블링이 AI 발전에 어떤 역할을 하는지에 대한 통찰을 제공합니다.
- "데이터 시각화 교과서" - 알베르토 카이로 지음
- 데이터를 효과적으로 이해하고 전달하기 위한 시각화 기법을 소개하며, 좋은 데이터 레이블링이 시각화의 질을 어떻게 높일 수 있는지 설명합니다.
이 책들은 데이터 레이블링과 관련된 지식뿐만 아니라, 데이터 과학, 머신러닝, 인공지능 전반에 대한 이해를 넓히는 데 도움이 될 것입니다.
#데이터 라벨링 #인공지능 #머신러닝 #딥러닝 #데이터분석 #AI교육 #데이터과학 #기술블로그 #테크트렌드 #컴퓨터비전 #자연어처리 #데이터 라벨링도구 #데이터 라벨링서비스 #데이터프로세싱 #AI발전 #데이터레이블링작업 #데이터품질 #기계학습 #인공지능트렌드 #스마트테크놀로지 #AI업데이트 #데이터 라벨링기술 #인공지능교육 #데이터 라벨링가이드 #AI연구
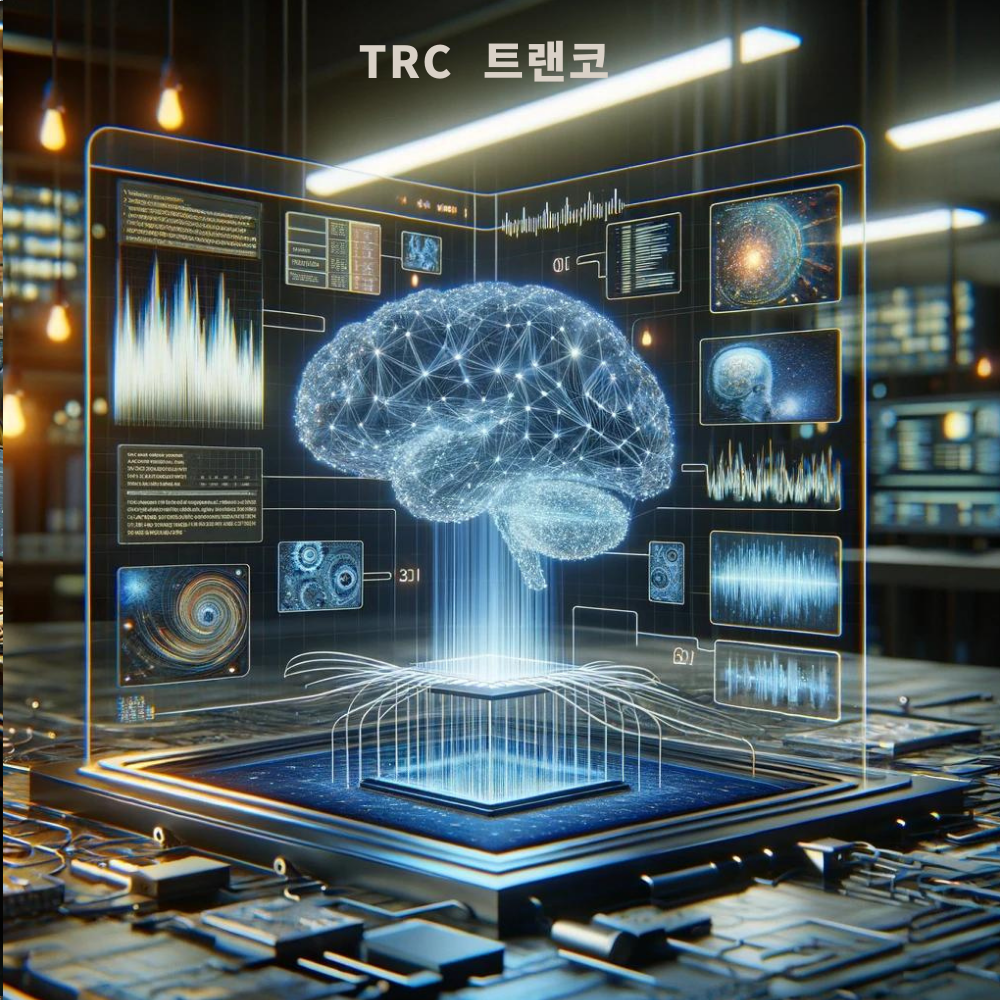
데이터 라벨링은
AI 시대의 숨은 영웅 같은 직업입니다.
눈에 띄지 않지만,
AI의 성장과 발전에 없어서는 안 될
중요한 역할을 하죠.
이 분야에 관심이 있으시다면,
지금 바로 도전해보는 것은 어떨까요?
누구나 시작할 수 있고,
AI의 미래를 함께 만들어가는 데
기여할 수 있는 멋진 기회랍니다!
여러분도 데이터 라벨링의 세계에 발을 들여놓아
AI의 미래를 함께 만들어가는 데 일조해보세요.
언제나처럼, 여러분의 도전을 응원합니다!

도구 및 플랫폼:
데이터 라벨링 작업을
더 쉽고 효율적으로 만들어주는
다양한 도구와 플랫폼이 있어요.
이들은 데이터 레이블러들이
수많은 데이터에 정확한 태그를 붙일 수 있도록 돕죠.
여기 몇 가지 인기 있는 도구와 플랫폼을 소개할게요:
1. Labelbox
Labelbox는 매우 인기 있는
데이터 라벨링 플랫폼 중 하나에요.
이미지, 비디오, 텍스트 등
다양한 유형의 데이터에
레이블을 붙일 수 있어요.
드래그 앤 드롭 인터페이스와 같은
직관적인 도구를 제공해서,
누구나 쉽게 사용할 수 있답니다.
팀워크와 협업을 위한
기능도 잘 갖추고 있어서,
여러 사람이 함께 작업하는
프로젝트에 아주 유용해요.
2. Annotator
Annotator는
주로 이미지와 텍스트 데이터에
초점을 맞춘 레이블링 도구에요.
사용자 친화적인 인터페이스를 제공하며,
간단한 마우스 클릭과 드래그로
데이터에 레이블을 붙일 수 있어요.
특히 이미지 데이터에 다양한 형태의 주석
(예: 박스, 폴리곤, 라인 등)을 추가할 수 있어서,
객체 감지나 세그먼테이션 작업에 적합해요.
3. Amazon Mechanical Turk (MTurk)
MTurk는 아마존이 운영하는
크라우드소싱 마켓플레이스로,
데이터 라벨링 작업을
'휴먼 인텔리전스 태스크(HIT)' 형태로 제공해요.
전 세계 수많은 '터커'들이
이 플랫폼을 통해
작은 작업들을 수행하며,
이를 통해 대규모 데이터 세트에
레이블을 붙일 수 있어요.
다양한 유형의 작업에 유연하게
적용될 수 있어서 매우 실용적이에요.
4. Prodi.gy
사용자가 자신만의
데이터 레이블링 워크플로우를
빠르게 구축할 수 있게 해주는 도구에요.
액티브 러닝 기능을 통해
가장 레이블링하기에
가치 있는 데이터를
우선적으로 제시하여,
시간과 자원을 효율적으로
사용할 수 있게 해줍니다.
특히 자연어 처리(NLP) 프로젝트에 아주 유용해요.
5. Label Studio
Label Studio는
다양한 데이터 소스와
유형을 지원하는
오픈 소스 데이터 라벨링 플랫폼이에요.
맞춤형 레이블링 인터페이스를 제공해서,
프로젝트의 특정 요구에 맞게 설정할 수 있어요.
이미지, 오디오, 텍스트 등
거의 모든 유형의 데이터에
레이블을 붙일 수 있으며,
사용자 친화적인 인터페이스로
누구나 쉽게 사용할 수 있어요.
이 도구들과 플랫폼들은
데이터 레이블링 작업을
훨씬 더 쉽고 효율적으로 만들어줍니다.
블로그 독자 여러분도
이 중 하나를 사용해보시면,
데이터 라벨링의 세계에
한 발짝 더 다가갈 수 있을 거예요!
Hello everyone!
Today, I'd like to talk about a profession that is quietly revolutionizing our daily lives.
It's the 'Data Labeling' expert,
who plays an important role behind the scenes of Artificial Intelligence (AI),
supporting AI quietly yet powerfully.
What is data labeling?
Simply put, data labeling is the process of attaching 'tags' or 'labels' to data
so that computers can understand it.
For example, tagging a cat in a photo as 'cat',
or attaching emotional labels like 'joy' or 'sadness' to sentences filled with emotions.
This labeled data serves as a textbook for AI to learn from.
Just like teaching a child the names of objects,
it helps teach AI about the world.
Why is it important?
In the world of AI and machine learning, data is like 'food'.
And the process that makes this food easy to 'digest' is
data labeling.
Accurate labeling determines the 'nutritional intake' of AI.
Incorrect labeling can lead the AI down the 'wrong path',
so the importance of this task cannot be overlooked.
How to become a data labeling expert?
Data labeling is a field that can be started without any special background knowledge.
However, it requires careful attention and accuracy.
A basic understanding of various data types, such as images, text, and audio, is needed,
and one must be proficient in using related tools and software.
Additionally, understanding the basic concepts of AI and machine learning can enable more effective labeling.
Future prospects?
As artificial intelligence continues to evolve,
the demand for data labeling is expected to increase.
With the adoption of AI expanding in various industries,
a career as a data labeling expert looks very promising.
In conclusion...
Data labeling is like the hidden hero of the AI era.
It plays a vital role in the growth and development of AI,
though it might not be visible.
If you're interested in this field,
why not take the challenge now?
It's a wonderful opportunity that anyone can start,
and contribute to shaping the future of AI!
Step into the world of data labeling and contribute to shaping the future of AI.
As always, we support your endeavors!
Please write in Korean language.
Tools and Platforms:
There are various tools and platforms that make data labeling work
easier and more efficient.
These help data labelers accurately tag numerous data.
Here are some popular tools and platforms:
- Labelbox
Labelbox is one of the most popular
data labeling platforms.
It allows you to label various types of data, such as images, videos, and texts.
It offers intuitive tools like a drag-and-drop interface,
making it easy for anyone to use.
It also has well-equipped features for teamwork and collaboration,
making it very useful for projects involving multiple people.
- Annotator
Annotator focuses mainly on image and text data labeling.
It provides a user-friendly interface,
allowing labels to be attached to data with simple mouse clicks and drags.
Especially for image data, it allows the addition of various forms of annotations
(e.g., boxes, polygons, lines), making it suitable for object detection or segmentation tasks.
- Amazon Mechanical Turk (MTurk)
MTurk is a crowdsourcing marketplace operated by Amazon,
offering data labeling tasks in the form of 'Human Intelligence Tasks (HITs)'.
Numerous 'Turkers' around the world
perform small tasks through this platform,
allowing labels to be attached to large data sets.
It is very practical as it can be flexibly applied to various types of tasks.
- Prodi.gy
This tool allows users to quickly build their own data labeling workflows.
Through active learning features,
it prioritizes the data most worth labeling,
enabling efficient use of time and resources.
It is particularly useful for Natural Language Processing (NLP) projects.
- Label Studio
Label Studio is an open-source data labeling platform
that supports various data sources and types.
It offers customizable labeling interfaces,
allowing settings to be adjusted to meet specific project needs.
It can label almost all types of data, including images, audio, and text,
with a user-friendly interface that anyone can easily use.
These tools and platforms make data labeling work
much easier and more efficient.
Blog readers, try using one of these,
and you'll be one step closer to the world of data label
'생활정보' 카테고리의 다른 글
"2024년 신용카드 세금 절세 꿀팁 대공개!" 연말정산 소득공제 환급 가족카드 재태크 노하우 (0) | 2024.02.05 |
---|---|
"온누리상품권 비밀: 당신이 몰랐던 세금 절세 전략!"설명절 상품권 한도 상향 (1) | 2024.02.04 |
"한강의 새로운 이동 수단, 리버버스 타고 출근해볼까?" (2) | 2024.01.22 |